This week’s maker is Leighton Hinkley, PhD, specialist in the Department of Radiology at UCSF. Let’s take a look at his project:
Q: What did you make?
I’ve worked with the UCSF Makers Lab since Fall 2023 to create a series of 3D-printed objects that act as part of a behavioral test in neurological disorders. This test requires participants to sort 3D shapes (spheres, cubes, etc.) solely by their sense of touch as quickly and accurately as possible. Patients with particular somatosensory or motor challenges as part of their disorder perform poorly on these tests, and tests like these can be really helpful for neuroscientists like me to measure the extent of these deficits. Because these types of tests are quite specialized and typically cannot be purchased from a regular supplier, the folks at the Makers Lab helped me take a spec sheet and turn them into a set of bespoke, 3D printed objects that work excellently in testing my patients.
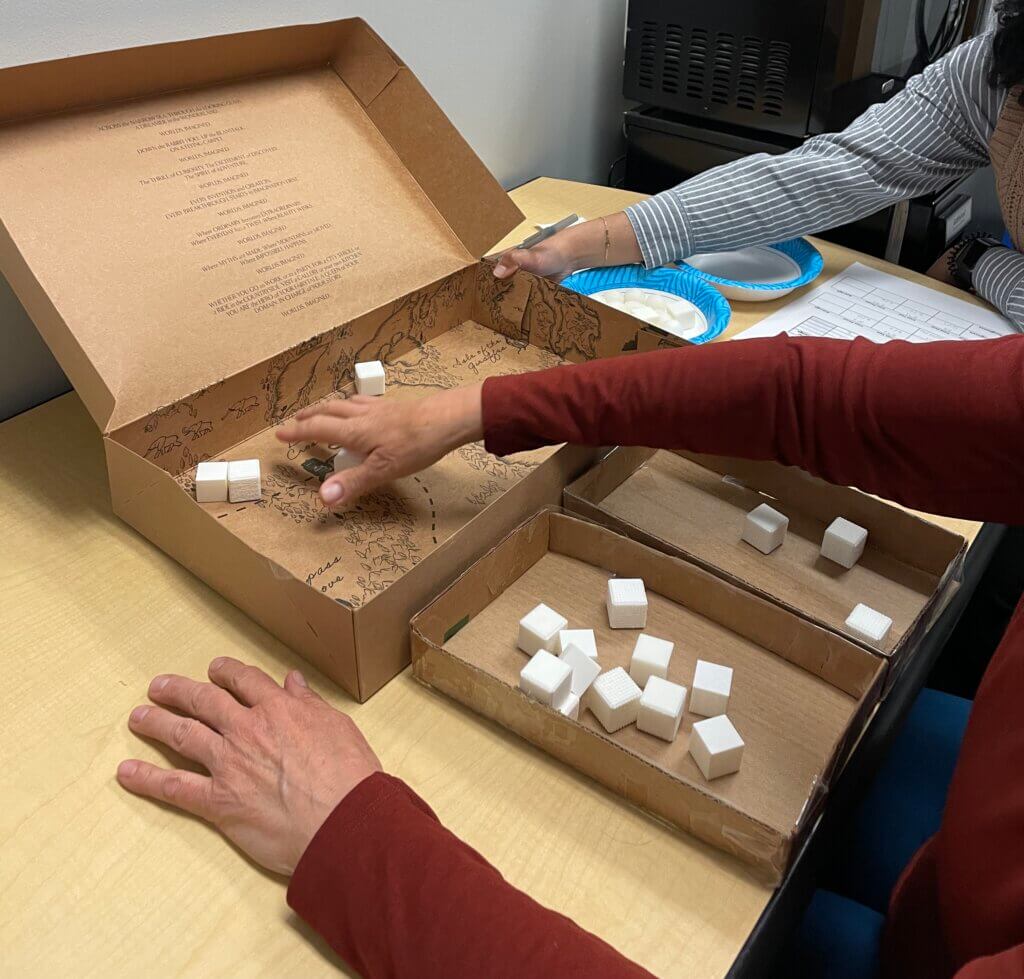
Q: Why did you want to make it?
I generously received a grant from the Dystonia Medical Research Foundation (DMRF) to study brain stimulation in what’s called focal dystonia — a hyperkinetic movement disorder where you have unwanted, sustained contractions of muscles. These contractions are uncomfortable and can make it difficult to perform certain motor tasks like speaking and writing. As part of our “outcome measures” to see if the brain stimulation was improving function in these patients, we needed a way to measure somatosensory (i.e. our sense of touch) skill in these patients.
One working hypothesis in dystonia is that the motor disability arises from tangled brain wiring in sensory systems. Typically, these folks will have challenges in telling certain shapes from each other (a process called stereognosis), and with detecting the roughness of tactile patterns (what we call graphesthesia). We need a test to measure both. A lot of tests that exist in physical therapy and neurology to measure these abilities can be either very expensive or difficult to obtain, and in some cases outdated. That’s why we reached out to the Makers Lab to see if they could take a set of instructions for a test in a previously published and well validated paper and use those instructions to print a test that we could directly use in the lab.


Left: figures from a paper by Hsu, Hsiu-Yun et al. Right: 3D modeled shapes created from specifications
Q: What was your process?
Like any good scientist, we consulted the literature as to what would be an optimal test for somatosensory function in hand dystonia. There are several publications that evaluate the validity of the test we needed, but these objects aren’t readily sold and often need to be custom built or crafted. Fortunately, the articles provided enough information (dimensions, resolution of gratings) that we were able to pass them on to Scott Drapeau, designer at the Makers Lab, and he assembled renders and printer files for the final product.
I then worked with Jenny Tai, Makers Lab engineer, to create prototypes. We first tried polylactic acid (PLA), a type of material commonly used in 3D printing that is cheap and lightweight, but unfortunately the quality of the prototypes didn’t meet our standards. These shapes need to be built with precision in order to ensure that every object is as similar as possible. Jenny suggested we switch to stereolithography (SLA) resin, which has a more durable build, and the final product turned out better than expected. The objects came out with the same grade of material that you’d commonly find in behavioral tests that would otherwise cost hundreds of dollars, or be out of circulation.
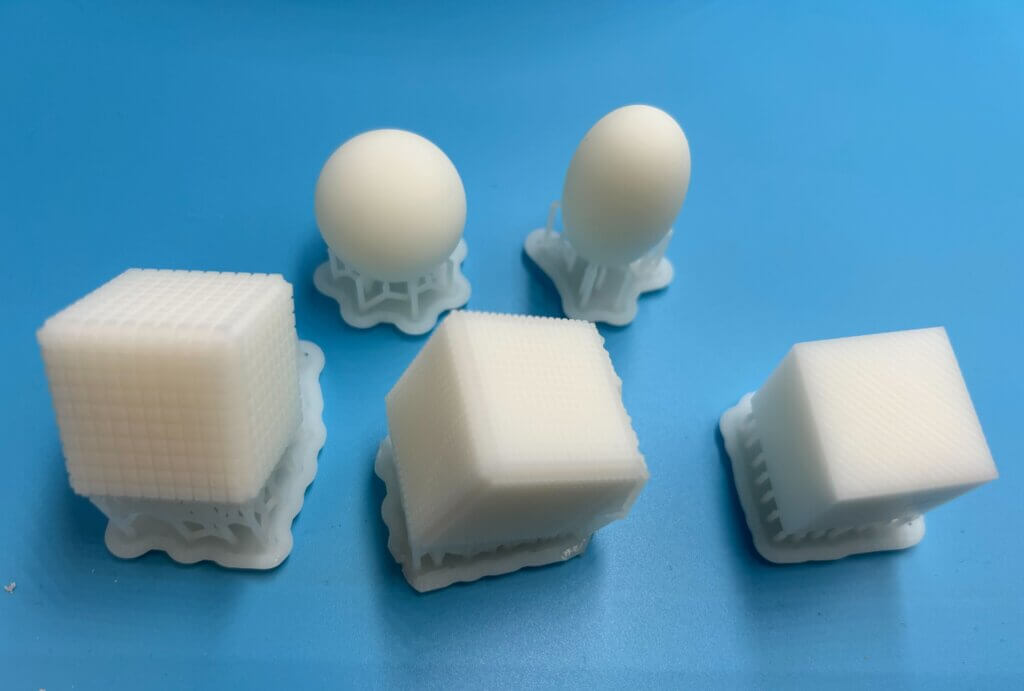
Q: What was the hardest part of the process?
Well, since this is supposed to be a sense of tactile ability — and some of the surfaces that come out of the printer can be a little rough — getting each object to its equal level of smoothness was a bit of a challenge. The folks at the UCSF Makers Lab provided some excellent guidance here, providing tools and strategies to make sure all of the objects were nearly identical.
Q: What was your favorite part of the process?
Honestly, being able to see the final product in a polished form was the most exciting to me. Often in research there are certain financial or practical obstacles that can get in the way of running the types of experiments you want to run, to test the hypotheses that are the most relevant interesting. Once Jenny presented me with the resin prints of the shapes I thought to myself “wow, we can do this…” and that is always a thrilling moment in the scientific method.
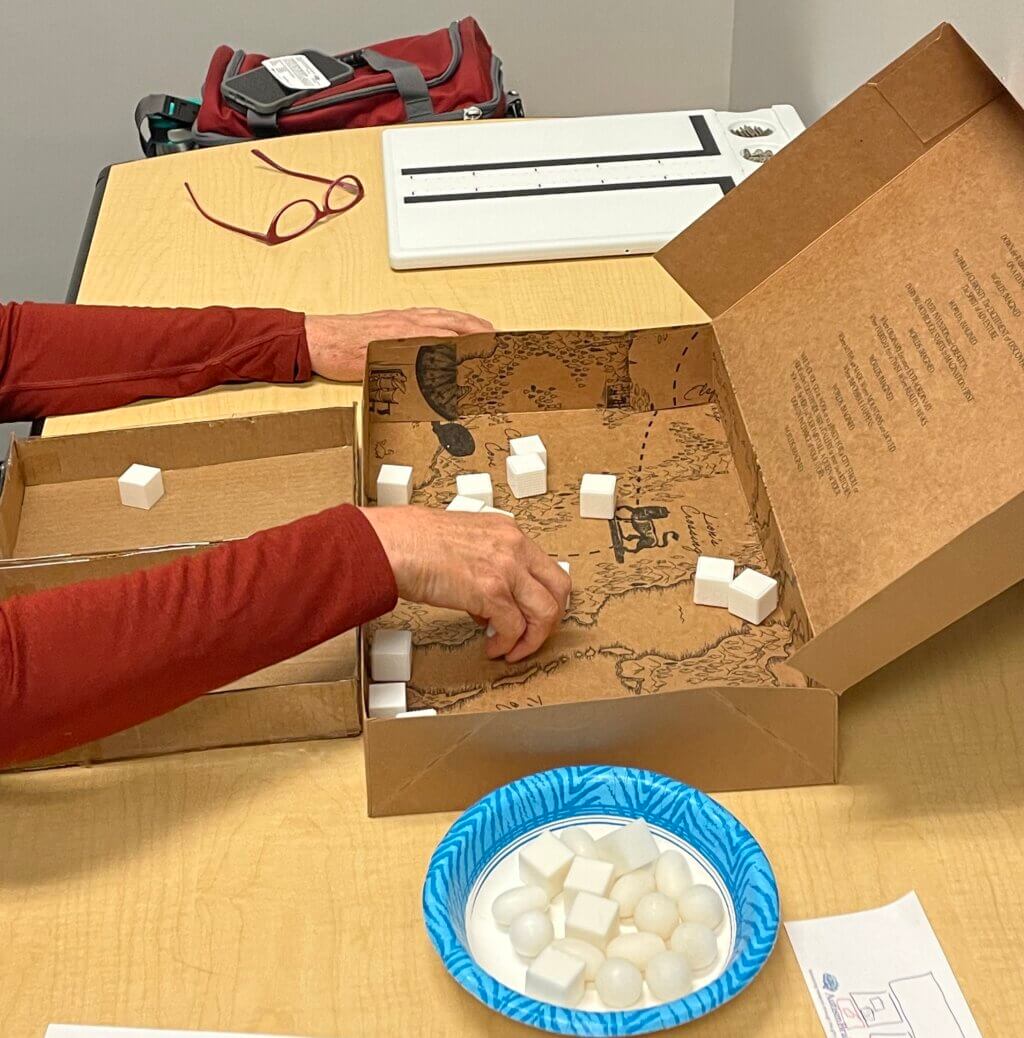
Q: How did this help make you a better scientist?
I think there are plenty of opportunities and resources provided by UCSF and the greater community that scientists here can tap into and use for their research — whether it is writing or AI assistance or fabrication resources like the Makers Lab. I don’t get to work with a lot of people in material science, so it was exciting to collaborate with them on the best build for our experimental design. They provided a lot of insight regarding the “nuts and bolts” of the build process that I would have not been exposed to otherwise. I’m thankful that the team at the Makers Lab was helpful and communicative, and I’ve been encouraging all of my collaborators to work with them whenever they need custom jobs.
Q: What do you want to make next?
I’m already working with Scott and Jenny for a case and mount of the existing somatosensory experiment to make the setup more professional, and to prevent the objects from rolling off of the workspace! I can already think of some other cool tests that we could print and use in our research, and I will be definitely reaching out to them for that.
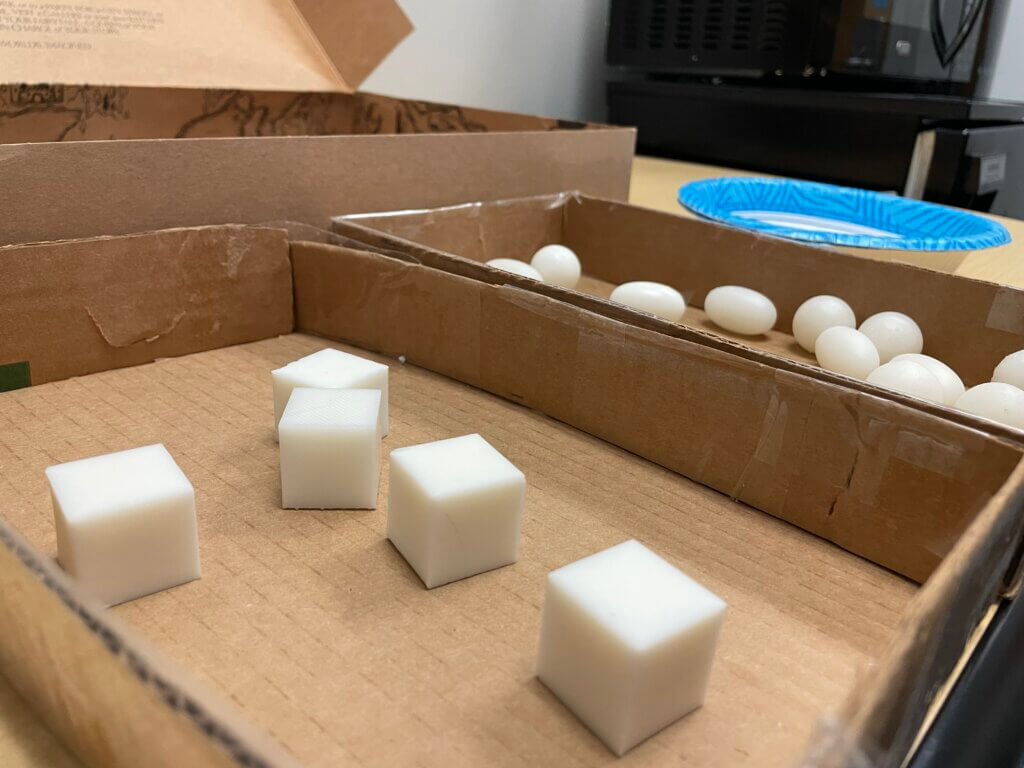